Evaluating AI Suitability: Inefficiencies, Hidden Costs and Avoidable Risks
Written by Stephen Clark | Business Architecture Practice Lead
If asked any of the following by a peer, a leader, or someone else, how would you respond?
-
- When does the inefficiency from query complexity outweigh perceived benefits of attempting to ask Large Language Models (LLMs) to help answer a question or analyze inputs?
- How do you evaluate trade-offs between complex prompt engineering investments and investments in expert input from human subject matter experts?
- How specific versus general should AI-supported technology solutions be, in order to justify what order-of-magnitude investments in off-the-shelf purchases, SaaS access, or internal engineering-driven custom solutions?
Logisolve has strong opinions about a range of answers to these questions. If you are attempting to mitigate risks, maximize investments and aspire to drive adoption of early applications of AI and advanced data science techniques and tools in your organization, read on. We want to talk with you about your challenges, priorities, and lessons learned.
With regard to the first question above, who do you work with to identify, analyze, and optimize the amount of forecast vs. actual electricity, water, real estate, hardware, and staff expenses you need to invest in or purchase access to in order to operate your ongoing versus ‘burst’ AI infrastructure?
Input Parameter | Description | Example Value |
Model Size | The size of the LLM in terms of parameters (e.g., billions of parameters) | 175 billion |
Compute Resources | The type and number of GPUs or TPUs used | 8 A100 GPUs |
Query Length | The average length of each query in tokens | 1000 tokens |
Query Volume | The number of queries processed per month | 1,000,000 queries |
Compute Time per Query | The average time taken to process each query (in seconds) | 0.5 seconds |
Energy Cost | The cost of electricity per kWh | $0.12 per kWh |
In the case of computing energy consumption costs, do you have more sophisticated estimation inputs than the following variables?
Parameter | Example Value |
Compute Resources | 8 A100 GPUs |
Compute Time | 139 hours |
Power Consumption per GPU | 400 watts |
Total Energy Consumption | 55.6 kWh |
Cost of Electricity | $0.12 per kWh |
Energy Cost | $6.67 |
Operational Overhead | 20% of compute cost |
Total Cost | $8.00 (approx.) |
How do you work with your product R&D, Technology, Supply Chain, Manufacturing, and Logistics colleagues to maximize chances of sustained, desired, AI usage versus accidentally large one-time, throw-away costs?
Regarding the second question at the start of this column, does your organization have an idea intake queue you can search and contribute to, in order to be part of planful, prioritized cross-functional continuous improvement processes?
If so, are there built-in decision-points where the organization asks questions like; 1) Do we have personnel who know answers to the questions we’re trying to address? or 2) Do our personnel with sufficient skills, expertise and interest ALSO have availability to help answer colleagues’ questions at the required frequency?
Trade-off considerations choosing between expert human knowledge and AI-provided answers could involve key considerations such as:
- Accuracy:
- Human Experts: Often have deep domain-specific knowledge and can provide highly accurate answers based on experience.
- AI: Can process vast amounts of data quickly and may identify patterns or insights that humans might miss. However, accuracy depends on the quality of the data and the model.
- Explainability:
- Human Experts: Can explain their reasoning and decision-making process, which is crucial for understanding and trust.
- AI: Often operates as a “black box,” making it difficult to understand how decisions are made. Some AI models, however, are designed to be more interpretable1.
- Speed and Scalability:
- Human Experts: Limited by time and availability. They may not be able to handle large volumes of queries quickly.
- AI: Can process and respond to queries at scale and in real-time, making it ideal for high-volume tasks.
- Cost:
- Human Experts: Can be expensive, especially for specialized knowledge.
- AI: Initial setup and training can be costly, but operational costs may be lower over time, especially for repetitive tasks.
- Contextual Understanding:
- Human Experts: Can understand and interpret nuanced contexts, cultural subtleties, and complex scenarios.
- AI: May struggle with context and nuance, especially in areas requiring deep understanding of human behavior or emotions.
- Adaptability:
- Human Experts: Can adapt to new information and changing circumstances quickly.
- AI: Requires retraining and updates to adapt to new data or changes in the environment.
- Ethical and Bias Considerations:
- Human Experts: Can consciously address ethical concerns and biases in their decision-making.
- AI: Can inadvertently perpetuate biases present in the training data, and ethical considerations must be carefully managed2.
- Reliability and Consistency:
- Human Experts: May have variability in their responses due to human factors like fatigue or personal biases.
- AI: Can provide consistent responses, but reliability depends on the robustness of the model and data quality.
Balancing these criteria depends on the specific use case and the importance of each factor.
Given the wide-array of supportive scenarios in which AI-supported solutions can contribute for individuals, teams, and larger combinations of collaborative communities, it is very interesting to analyze what patterns in solution design, implementation and ongoing support/maintenance make sense for what sized investments.
Has your organization focused on role-focused effectiveness improvements or multi-role enabling mechanisms, or both? If both, what percentage in which category?
What combination of justification classifications is your organization using to evaluate success? Are assessments of moving faster, smarter, more easily, more profitably, with less risk or other qualitative or quantitative success criteria the dominant combinations?
- Business Value:
- Cost Reduction: Will AI help reduce operational costs?
- Revenue Generation: Can AI create new revenue streams or enhance existing ones?
- Efficiency Improvement: Will AI streamline processes and improve productivity?
- Technical Feasibility:
- Data Availability: Is there sufficient and high-quality data to train the AI models?
- Infrastructure: Do you have the necessary hardware and software infrastructure?
- Integration: Can AI be integrated with existing systems and workflows?
- Strategic Alignment:
- Business Goals: Does the AI solution align with the company’s strategic objectives?
- Competitive Advantage: Will AI provide a competitive edge in the market?
- Scalability: Can the AI solution scale with the business growth?
- Risk Management:
- Ethical Considerations: Are there ethical implications, such as bias or fairness, that need to be addressed?
- Compliance: Does the AI solution comply with relevant regulations and standards?
- Security: Are there measures in place to protect data and AI systems from cyber threats?
- Operational Impact:
- Change Management: Is there a plan for managing the change within the organization?
- Training and Support: Will employees receive the necessary training and support to work with AI?
- Maintenance: Are there resources allocated for the ongoing maintenance and updates of the AI system?
- Performance Metrics:
- KPIs: What key performance indicators (KPIs) will be used to measure the success of the AI implementation?
- ROI: What is the expected return on investment (ROI) from the AI solution?
With regard to the third question at the start of this column, what is your primary point-of-view, when you approach discussions and decisions related to AI?
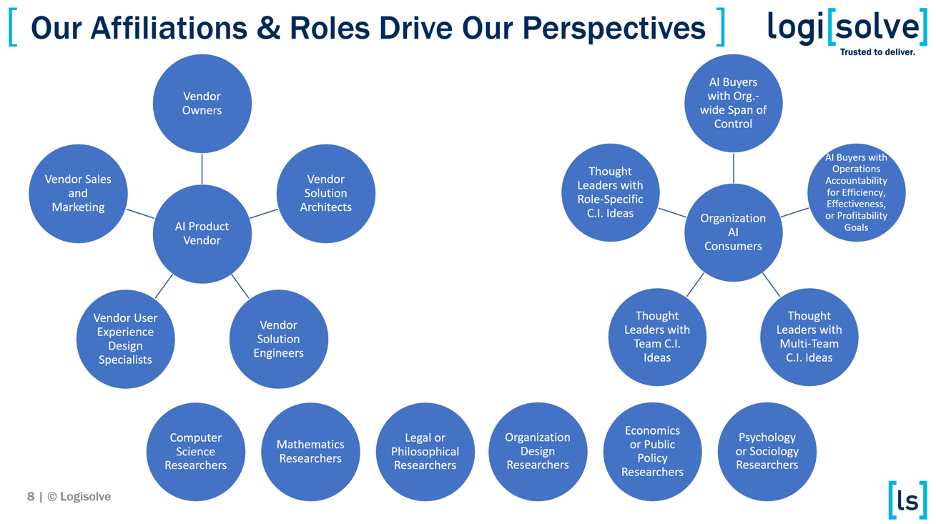
Depending upon your answer, and it could encompass multiple roles on one or more of the ‘flowers,’ what aspects of engineering or adoption complexity are you focused most?
-
- Do you know about machine learning operations (MLOps)?
- How many providers of different kinds of MLOps is your organization evaluating or using?
- If you are not evaluating how to operationalize your AI design, acquisition, integration, testing and adoption activities, why not?
As we quickly approach February, we share with you this invitation. Logisolve is currently helping clients address the following dimensions of AI evaluation, acquisition, integration and adoption success planning and management.
-
- 6 Dimensions of Observability
- 10 Dimensions of Risk Mitigation Priority Setting in Legal, Ethical, Moral contexts
- 11 Dimensions of Implementation Robustness
- 10 Dimensions of Technical & Cultural Challenges
- 14 Dimensions of User Experience Measurement and Metrics Evaluation
If you want to learn more, please reach out to SClark@Logisolve.com.
Volunteer with Logisolve in 2025!
Logisolve is proud to continue its partnership with Flourish Food Market by hosting monthly volunteer opportunities. Join us on the first Wednesday of each month for one of two shifts: 11:00 AM–1:00 PM or 1:00 PM–3:30 PM. If you’re interested in participating, please reach out to Ron Kimlinger at rkimlinger@logisolve.com. We are excited to strengthen this meaningful collaboration with the Center of Belonging organization and make a positive impact together.
Explore Career Opportunities with Logisolve!
Looking for your next opportunity? At Logisolve, we have a variety of contract and contract-to-hire roles available across all our capabilities. If you’re interested or know someone who is, please reach out to us at referrals@logisolve.com. We’d love to connect!